Lecture 3: Class demo#
Imports, Announcements, LOs#
Imports#
# import the libraries
import os
import sys
sys.path.append("../code/.")
from plotting_functions import *
from utils import *
import matplotlib.pyplot as plt
import numpy as np
import pandas as pd
%matplotlib inline
pd.set_option("display.max_colwidth", 200)
Data#
Let’s bring back King County housing sale prediction data from the course introduction video. You can download the data from here.
housing_df = pd.read_csv('../data/kc_house_data.csv')
housing_df
id | date | price | bedrooms | bathrooms | sqft_living | sqft_lot | floors | waterfront | view | ... | grade | sqft_above | sqft_basement | yr_built | yr_renovated | zipcode | lat | long | sqft_living15 | sqft_lot15 | |
---|---|---|---|---|---|---|---|---|---|---|---|---|---|---|---|---|---|---|---|---|---|
0 | 7129300520 | 20141013T000000 | 221900.0 | 3 | 1.00 | 1180 | 5650 | 1.0 | 0 | 0 | ... | 7 | 1180 | 0 | 1955 | 0 | 98178 | 47.5112 | -122.257 | 1340 | 5650 |
1 | 6414100192 | 20141209T000000 | 538000.0 | 3 | 2.25 | 2570 | 7242 | 2.0 | 0 | 0 | ... | 7 | 2170 | 400 | 1951 | 1991 | 98125 | 47.7210 | -122.319 | 1690 | 7639 |
2 | 5631500400 | 20150225T000000 | 180000.0 | 2 | 1.00 | 770 | 10000 | 1.0 | 0 | 0 | ... | 6 | 770 | 0 | 1933 | 0 | 98028 | 47.7379 | -122.233 | 2720 | 8062 |
3 | 2487200875 | 20141209T000000 | 604000.0 | 4 | 3.00 | 1960 | 5000 | 1.0 | 0 | 0 | ... | 7 | 1050 | 910 | 1965 | 0 | 98136 | 47.5208 | -122.393 | 1360 | 5000 |
4 | 1954400510 | 20150218T000000 | 510000.0 | 3 | 2.00 | 1680 | 8080 | 1.0 | 0 | 0 | ... | 8 | 1680 | 0 | 1987 | 0 | 98074 | 47.6168 | -122.045 | 1800 | 7503 |
... | ... | ... | ... | ... | ... | ... | ... | ... | ... | ... | ... | ... | ... | ... | ... | ... | ... | ... | ... | ... | ... |
21608 | 263000018 | 20140521T000000 | 360000.0 | 3 | 2.50 | 1530 | 1131 | 3.0 | 0 | 0 | ... | 8 | 1530 | 0 | 2009 | 0 | 98103 | 47.6993 | -122.346 | 1530 | 1509 |
21609 | 6600060120 | 20150223T000000 | 400000.0 | 4 | 2.50 | 2310 | 5813 | 2.0 | 0 | 0 | ... | 8 | 2310 | 0 | 2014 | 0 | 98146 | 47.5107 | -122.362 | 1830 | 7200 |
21610 | 1523300141 | 20140623T000000 | 402101.0 | 2 | 0.75 | 1020 | 1350 | 2.0 | 0 | 0 | ... | 7 | 1020 | 0 | 2009 | 0 | 98144 | 47.5944 | -122.299 | 1020 | 2007 |
21611 | 291310100 | 20150116T000000 | 400000.0 | 3 | 2.50 | 1600 | 2388 | 2.0 | 0 | 0 | ... | 8 | 1600 | 0 | 2004 | 0 | 98027 | 47.5345 | -122.069 | 1410 | 1287 |
21612 | 1523300157 | 20141015T000000 | 325000.0 | 2 | 0.75 | 1020 | 1076 | 2.0 | 0 | 0 | ... | 7 | 1020 | 0 | 2008 | 0 | 98144 | 47.5941 | -122.299 | 1020 | 1357 |
21613 rows × 21 columns
Exploratory Data Analysis#
Is this a classification problem or a regression problem?
# How many data points do we have?
n = housing_df.shape[0]
n
21613
# What are the columns in the dataset?
housing_df.columns
Index(['id', 'date', 'price', 'bedrooms', 'bathrooms', 'sqft_living',
'sqft_lot', 'floors', 'waterfront', 'view', 'condition', 'grade',
'sqft_above', 'sqft_basement', 'yr_built', 'yr_renovated', 'zipcode',
'lat', 'long', 'sqft_living15', 'sqft_lot15'],
dtype='object')
# Do we need to keep all the columns?
X = housing_df.drop(columns=['id', 'date', 'zipcode', 'price'])
y = housing_df['price']
Let’s explore some features. Let’s try the describe()
method
X.describe()
bedrooms | bathrooms | sqft_living | sqft_lot | floors | waterfront | view | condition | grade | sqft_above | sqft_basement | yr_built | yr_renovated | lat | long | sqft_living15 | sqft_lot15 | |
---|---|---|---|---|---|---|---|---|---|---|---|---|---|---|---|---|---|
count | 21613.000000 | 21613.000000 | 21613.000000 | 2.161300e+04 | 21613.000000 | 21613.000000 | 21613.000000 | 21613.000000 | 21613.000000 | 21613.000000 | 21613.000000 | 21613.000000 | 21613.000000 | 21613.000000 | 21613.000000 | 21613.000000 | 21613.000000 |
mean | 3.370842 | 2.114757 | 2079.899736 | 1.510697e+04 | 1.494309 | 0.007542 | 0.234303 | 3.409430 | 7.656873 | 1788.390691 | 291.509045 | 1971.005136 | 84.402258 | 47.560053 | -122.213896 | 1986.552492 | 12768.455652 |
std | 0.930062 | 0.770163 | 918.440897 | 4.142051e+04 | 0.539989 | 0.086517 | 0.766318 | 0.650743 | 1.175459 | 828.090978 | 442.575043 | 29.373411 | 401.679240 | 0.138564 | 0.140828 | 685.391304 | 27304.179631 |
min | 0.000000 | 0.000000 | 290.000000 | 5.200000e+02 | 1.000000 | 0.000000 | 0.000000 | 1.000000 | 1.000000 | 290.000000 | 0.000000 | 1900.000000 | 0.000000 | 47.155900 | -122.519000 | 399.000000 | 651.000000 |
25% | 3.000000 | 1.750000 | 1427.000000 | 5.040000e+03 | 1.000000 | 0.000000 | 0.000000 | 3.000000 | 7.000000 | 1190.000000 | 0.000000 | 1951.000000 | 0.000000 | 47.471000 | -122.328000 | 1490.000000 | 5100.000000 |
50% | 3.000000 | 2.250000 | 1910.000000 | 7.618000e+03 | 1.500000 | 0.000000 | 0.000000 | 3.000000 | 7.000000 | 1560.000000 | 0.000000 | 1975.000000 | 0.000000 | 47.571800 | -122.230000 | 1840.000000 | 7620.000000 |
75% | 4.000000 | 2.500000 | 2550.000000 | 1.068800e+04 | 2.000000 | 0.000000 | 0.000000 | 4.000000 | 8.000000 | 2210.000000 | 560.000000 | 1997.000000 | 0.000000 | 47.678000 | -122.125000 | 2360.000000 | 10083.000000 |
max | 33.000000 | 8.000000 | 13540.000000 | 1.651359e+06 | 3.500000 | 1.000000 | 4.000000 | 5.000000 | 13.000000 | 9410.000000 | 4820.000000 | 2015.000000 | 2015.000000 | 47.777600 | -121.315000 | 6210.000000 | 871200.000000 |
# What are the value counts of the `waterfront` feature?
X['waterfront'].value_counts()
waterfront
0 21450
1 163
Name: count, dtype: int64
# What are the value_counts of `yr_renovated` feature?
X['yr_renovated'].value_counts()
yr_renovated
0 20699
2014 91
2013 37
2003 36
2005 35
...
1951 1
1959 1
1948 1
1954 1
1944 1
Name: count, Length: 70, dtype: int64
Many opportunities to clean the data but we’ll stop here.
Baseline model#
# Train a DummyRegressor model
from sklearn.dummy import DummyRegressor # Import DummyRegressor
# Create a class object for the sklearn model.
dummy = DummyRegressor()
# fit the dummy regressor
dummy.fit(X, y)
# score the model
dummy.score(X, y)
0.0
# predict on X using the model
dummy.predict(X)
array([540088.14176653, 540088.14176653, 540088.14176653, ...,
540088.14176653, 540088.14176653, 540088.14176653])
Decision tree model#
# Train a decision tree model
from sklearn.tree import DecisionTreeRegressor # Import DecisionTreeRegressor
# Create a class object for the sklearn model.
dt = DecisionTreeRegressor(random_state=123)
# fit the decision tree regressor
dt.fit(X, y)
# score the model
dt.score(X, y)
0.9991338290544213
We are getting a perfect accuracy. Should we be happy with this model and deploy it? Why or why not?
What’s the depth of this model?
dt.get_depth()
38
Data splitting#
Let’s split the data and
Train on the train split
Score on the test split
# Split the data
from sklearn.model_selection import train_test_split
X_train, X_test, y_train, y_test = train_test_split(X, y, test_size=0.2, random_state=123)
# Instantiate a class object
dt = DecisionTreeRegressor(random_state=123)
# Train a decision tree on X_train, y_train
dt.fit(X_train, y_train)
# Score on the train set
dt.score(X_train, y_train)
0.9994394006711425
# Score on the test set
dt.score(X_test, y_test)
0.719915905190645
Activity: Discuss the following questions in your group#
Why is there a large gap between train and test scores?
What would be the effect of increasing or decreasing
test_size
?Why are we setting the
random_state
? Is it a good idea to try a bunch of values for therandom_state
and pick the one which gives the best scores?Would it be possible to further improve the scores?
Let’s try out different depths.
max_depth= 1
dt = DecisionTreeRegressor(max_depth=1, random_state=123)
dt.fit(X_train, y_train)
DecisionTreeRegressor(max_depth=1, random_state=123)In a Jupyter environment, please rerun this cell to show the HTML representation or trust the notebook.
On GitHub, the HTML representation is unable to render, please try loading this page with nbviewer.org.
DecisionTreeRegressor(max_depth=1, random_state=123)
# Visualize your decision stump
from sklearn.tree import plot_tree
plot_tree(dt, feature_names = X.columns.tolist(), impurity=False, filled=True);
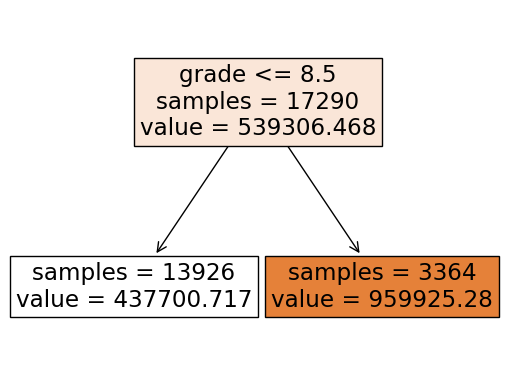
dt.score(X_train, y_train) # Score on the train set
0.3209427041566191
dt.score(X_test, y_test) # Score on the test set
0.31767136668453344
How do these scores compare to the previous scores?
Let’s try depth 10.
dt = DecisionTreeRegressor(max_depth=10, random_state=123) # max_depth= 10
dt.fit(X_train, y_train)
DecisionTreeRegressor(max_depth=10, random_state=123)In a Jupyter environment, please rerun this cell to show the HTML representation or trust the notebook.
On GitHub, the HTML representation is unable to render, please try loading this page with nbviewer.org.
DecisionTreeRegressor(max_depth=10, random_state=123)
dt.score(X_train, y_train) # Score on the train set
0.9108334653214172
dt.score(X_test, y_test) # Score on the test set
0.7728396574320712
Any improvements? Which depth should we pick?
Single validation set#
We are using the test data again and again. How about creating a validation set to pick the right depth and assessing the final model on the test set?
# Create a validation set
X_tr, X_valid, y_tr, y_valid = train_test_split(X_train, y_train, test_size=0.2, random_state=123)
tr_scores = []
valid_scores = []
depths = np.arange(1, 35, 2)
for depth in depths:
# Create and fit a decision tree model for the given depth
dt = DecisionTreeRegressor(max_depth=depth, random_state=123)
dt.fit(X_tr, y_tr)
# Calculate and append r2 scores on the training and validation sets
tr_scores.append(dt.score(X_tr, y_tr))
valid_scores.append(dt.score(X_valid, y_valid))
results_single_valid_df = pd.DataFrame({"train_score": tr_scores,
"valid_score": valid_scores},index = depths)
results_single_valid_df
train_score | valid_score | |
---|---|---|
1 | 0.319559 | 0.326616 |
3 | 0.603739 | 0.555180 |
5 | 0.754938 | 0.677567 |
7 | 0.833913 | 0.737285 |
9 | 0.890456 | 0.763480 |
11 | 0.931896 | 0.790521 |
13 | 0.963024 | 0.769030 |
15 | 0.981643 | 0.752728 |
17 | 0.991810 | 0.735637 |
19 | 0.996424 | 0.745925 |
21 | 0.998370 | 0.734048 |
23 | 0.999213 | 0.741060 |
25 | 0.999480 | 0.722873 |
27 | 0.999544 | 0.723951 |
29 | 0.999558 | 0.734986 |
31 | 0.999562 | 0.724068 |
33 | 0.999567 | 0.724410 |
results_single_valid_df[['train_score', 'valid_score']].plot(ylabel='r2 scores');
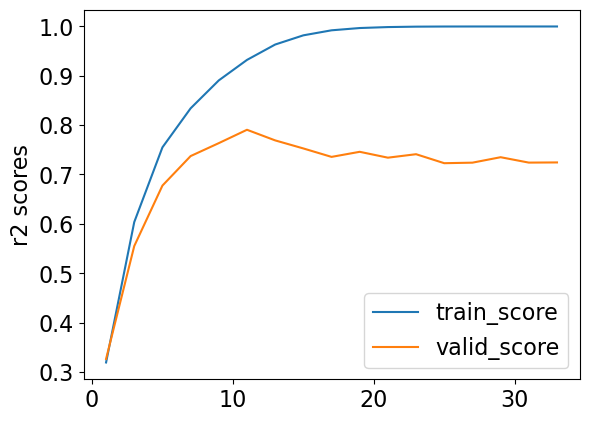
What depth gives the “best” validation score?
best_depth = results_single_valid_df.index.values[np.argmax(results_single_valid_df['valid_score'])]
best_depth
11
Let’s assess the best model on the test set.
test_model = DecisionTreeRegressor(max_depth=best_depth, random_state=123)
test_model.fit(X_train, y_train)
test_model.score(X_test, y_test)
0.7784948928666875
How do the test scores compare to the validation scores?
Can we have a more robust estimate of the test score?
Cross-validation#
depths = np.arange(1, 35, 2)
cv_train_scores = []
cv_valid_scores = []
for depth in depths:
# Create and fit a decision tree model for the given depth
dt = DecisionTreeRegressor(max_depth = depth, random_state=123)
# Carry out cross-validation
scores = cross_validate(dt, X_train, y_train, return_train_score=True)
cv_train_scores.append(scores['train_score'].mean())
cv_valid_scores.append(scores['test_score'].mean())
results_df = pd.DataFrame({"train_score": cv_train_scores,
"valid_score": cv_valid_scores
},
index=depths
)
results_df
train_score | valid_score | |
---|---|---|
1 | 0.321050 | 0.322465 |
3 | 0.603243 | 0.559284 |
5 | 0.752169 | 0.688484 |
7 | 0.835876 | 0.758259 |
9 | 0.894960 | 0.768184 |
11 | 0.938201 | 0.772185 |
13 | 0.966812 | 0.760966 |
15 | 0.983340 | 0.754620 |
17 | 0.992220 | 0.730025 |
19 | 0.996487 | 0.722803 |
21 | 0.998440 | 0.726659 |
23 | 0.999178 | 0.730704 |
25 | 0.999438 | 0.711356 |
27 | 0.999518 | 0.721917 |
29 | 0.999539 | 0.729374 |
31 | 0.999545 | 0.740319 |
33 | 0.999546 | 0.706489 |
results_df[['train_score', 'valid_score']].plot(ylabel='r2 score', title='Housing price prediction depth vs. r2 score');
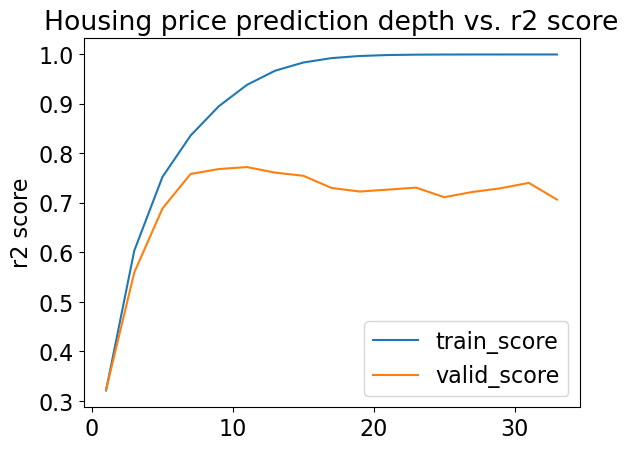
What’s the “best” depth with cross-validation?
best_depth = results_df.index.values[np.argmax(results_df['valid_score'])]
best_depth
11
Discuss the following questions in your group#
For which depth(s) we are underfitting? How about overfitting?
Above we are picking the depth which gives us the best cross-validation score. Is it always a good idea to pick such a depth? What if you have a much simpler model (smaller
max_depth
), which gives us almost the same CV scores?If we care about the test scores in the end, why don’t we use it in training?
Do you trust our hyperparameter optimization? In other words, do you believe that we have found the best possible depth?
Assessing on the test set#
dt_final = DecisionTreeRegressor(max_depth=best_depth, random_state=123)
dt_final.fit(X_train, y_train)
dt_final.score(X_train, y_train)
0.9308647034083802
dt_final.score(X_test, y_test)
0.7784948928666875
How do these scores compare to the scores when we used a single validation set?
Learned model#
#What's the depth of the model?
dt_final.get_depth()
11
plot_tree(dt_final, feature_names = X_train.columns.tolist(), impurity=False, filled=True);
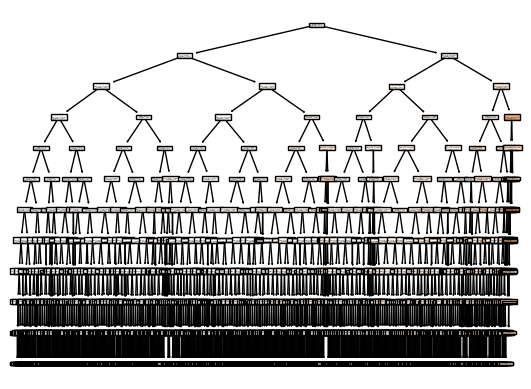
# Which features are the most important ones?
dt_final.feature_importances_
array([0.00080741, 0.00327551, 0.25123925, 0.01808825, 0.00079645,
0.03213916, 0.01190633, 0.00106308, 0.36400802, 0.02313684,
0.00295235, 0.01209545, 0.00064647, 0.17216105, 0.06835056,
0.02416048, 0.01317334])
Let’s examine feature importances.
df = pd.DataFrame(
data = {
"features": dt_final.feature_names_in_,
"feature_importances": dt_final.feature_importances_
}
)
df.sort_values("feature_importances", ascending=False)
features | feature_importances | |
---|---|---|
8 | grade | 0.364008 |
2 | sqft_living | 0.251239 |
13 | lat | 0.172161 |
14 | long | 0.068351 |
5 | waterfront | 0.032139 |
15 | sqft_living15 | 0.024160 |
9 | sqft_above | 0.023137 |
3 | sqft_lot | 0.018088 |
16 | sqft_lot15 | 0.013173 |
11 | yr_built | 0.012095 |
6 | view | 0.011906 |
1 | bathrooms | 0.003276 |
10 | sqft_basement | 0.002952 |
7 | condition | 0.001063 |
0 | bedrooms | 0.000807 |
4 | floors | 0.000796 |
12 | yr_renovated | 0.000646 |