Appendix B: Multi-class, meta-strategies#
So far we have been talking about binary classification
Can we use these classifiers when there are more than two classes?
“ImageNet” computer vision competition, for example, has 1000 classes
Can we use decision trees or KNNs for multi-class classification?
What about logistic regression and Linear SVMs?
Many linear classification models don’t extend naturally to the multiclass case.
A common technique is to reduce multiclass classication into several instances of binary classification problems.
Two kind of “hacky” ways to reduce multi-class classification into binary classification:
the one-vs.-rest approach
the one-vs.-one approach
One vs. Rest#
1v{2,3}, 2v{1,3}, 3v{1,2}
Learn a binary model for each class which tries to separate that class from all of the other classes.
If you have \(k\) classes, it’ll train \(k\) binary classifiers, one for each class.
Trained on imbalanced datasets containing all examples.
Given a test point, get scores from all binary classifiers (e.g., raw scores for logistic regression).
The classifier which has the highest score for this class “wins” and that’s going to be the prediction for this class.
Since we have one binary classifier per class, we have coefficients per feature and an intercept for each class.
Note
Note that there is also a multinomial logistic regression also called as the maxent classifier. This is different than the above multi-class meta strategies. More on this in DSCI 573.
Let’s create some synthetic data with two features and three classes.
import mglearn
from sklearn.datasets import make_blobs
X, y = make_blobs(centers=3, n_samples=120, random_state=42)
X_train, X_test, y_train, y_test = train_test_split(
X, y, test_size=0.2, random_state=123
)
mglearn.discrete_scatter(X_train[:, 0], X_train[:, 1], y_train)
plt.xlabel("Feature 0")
plt.ylabel("Feature 1")
plt.legend(["Class 0", "Class 1", "Class 2"]);
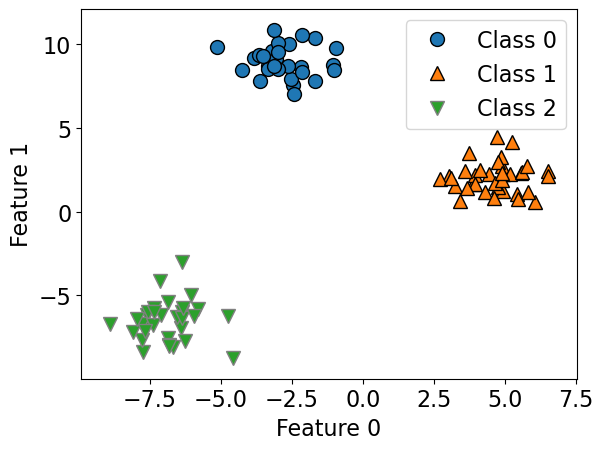
lr = LogisticRegression(max_iter=2000, multi_class="ovr")
lr.fit(X_train, y_train)
print("Coefficient shape: ", lr.coef_.shape)
print("Intercept shape: ", lr.intercept_.shape)
Coefficient shape: (3, 2)
Intercept shape: (3,)
/Users/kvarada/miniforge3/envs/571/lib/python3.12/site-packages/sklearn/linear_model/_logistic.py:1256: FutureWarning: 'multi_class' was deprecated in version 1.5 and will be removed in 1.7. Use OneVsRestClassifier(LogisticRegression(..)) instead. Leave it to its default value to avoid this warning.
warnings.warn(
This learns three binary linear models.
So we have coefficients for two features for each of these three linear models.
Also we have three intercepts, one for each class.
# Function definition in code/plotting_functions.py
plot_multiclass_lr_ovr(lr, X_train, y_train, 3)
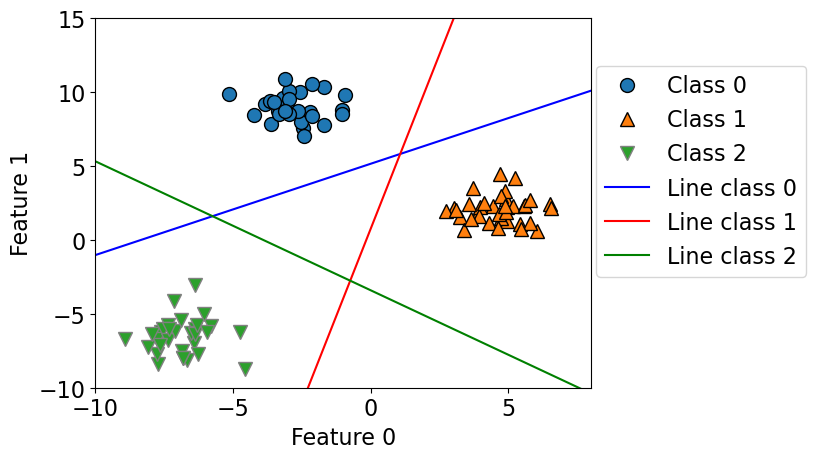
How would you classify the following points?
The answer is pick the class with the highest value for the classification formula.
test_points = [[-4.0, 12], [-2, 0.0], [-8, 3.0], [4, 8.5], [0, -7]]
plot_multiclass_lr_ovr(lr, X_train, y_train, 3, test_points)
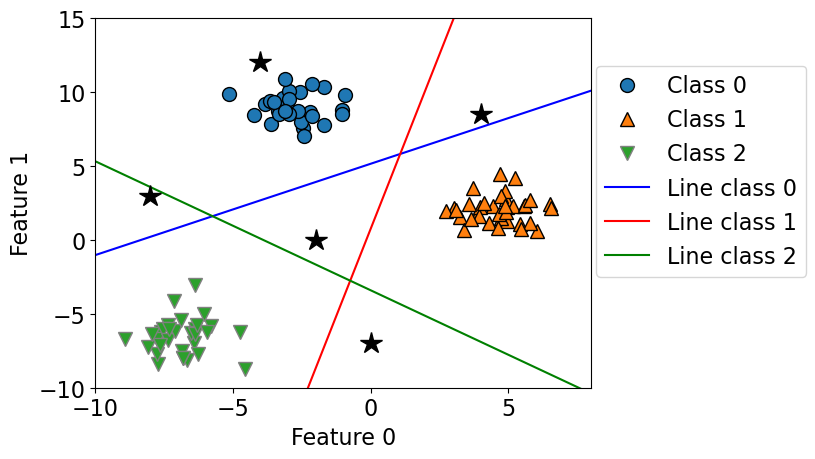
plot_multiclass_lr_ovr(lr, X_train, y_train, 3, test_points, decision_boundary=True)
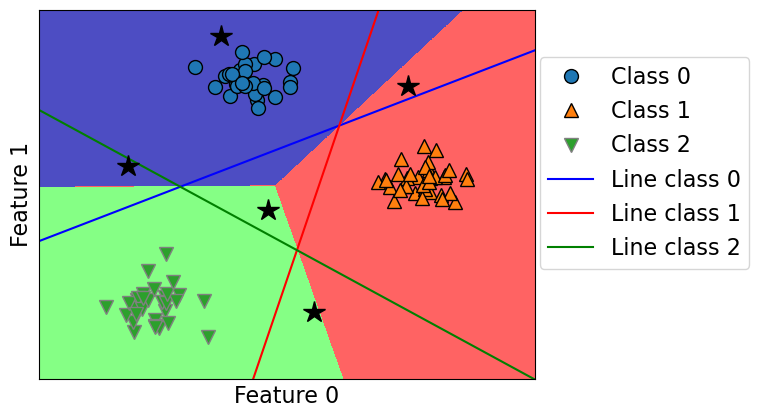
Let’s calculate the raw scores for a test point.
test_points[4]
[0, -7]
lr.coef_
array([[-0.65125032, 1.05350962],
[ 1.35375221, -0.2865025 ],
[-0.63316788, -0.7250894 ]])
lr.intercept_
array([-5.42541681, 0.21616484, -2.47242662])
test_points[4]@lr.coef_.T + lr.intercept_
array([-12.79998417, 2.22168237, 2.60319915])
lr.classes_
array([0, 1, 2])
Class 1 and 2 seems to have similar scores, which makes sense because the point is close to the border. But it’s in the green region because Class 2 score is the highest.
lr.predict_proba([test_points[4]])
array([[1.50596432e-06, 4.92120500e-01, 5.07877994e-01]])
One Vs. One approach#
Build a binary model for each pair of classes.
1v2, 1v3, 2v3
Trains n * (n-1)/2 binary classifiers
Trained on relatively balanced subsets
One Vs. One prediction#
Apply all of the classifiers on the test example.
Count how often each class was predicted.
Predict the class with most votes.
Using OVR and OVO as wrappers#
You can use these strategies as meta-strategies for any binary classifiers.
When do we use
OneVsRestClassifier
andOneVsOneClassifier
It’s not that likely for you to need
OneVsRestClassifier
orOneVsOneClassifier
because most of the methods you’ll use will have native multi-class support.However, it’s good to know in case you ever need to extend a binary classifier (perhaps one you’ve implemented on your own).
from sklearn.multiclass import OneVsOneClassifier, OneVsRestClassifier
# Let's examine the time taken by OneVsRestClassifier and OneVsOneClassifier
# generate blobs with fixed random generator
X_multi, y_multi = make_blobs(n_samples=1000, centers=20, random_state=300)
X_train_multi, X_test_multi, y_train_multi, y_test_multi = train_test_split(
X_multi, y_multi
)
mglearn.discrete_scatter(X_train_multi[:, 0], X_train_multi[:, 1], y_train_multi, s=6);
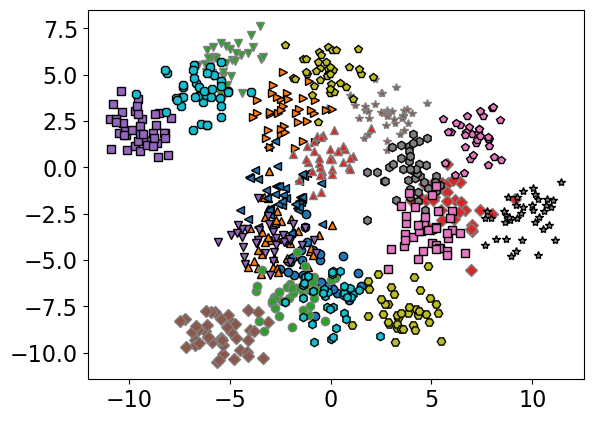
model = OneVsOneClassifier(LogisticRegression())
%timeit model.fit(X_train_multi, y_train_multi);
print("With OVO wrapper")
print(model.score(X_train_multi, y_train_multi))
print(model.score(X_test_multi, y_test_multi))
148 ms ± 7.84 ms per loop (mean ± std. dev. of 7 runs, 10 loops each)
With OVO wrapper
0.792
0.792
model = OneVsRestClassifier(LogisticRegression())
%timeit model.fit(X_train_multi, y_train_multi);
print("With OVR wrapper")
print(model.score(X_train_multi, y_train_multi))
print(model.score(X_test_multi, y_test_multi))
22 ms ± 490 μs per loop (mean ± std. dev. of 7 runs, 10 loops each)
With OVR wrapper
0.7266666666666667
0.68
As expected OVO takes more time compared to OVR
Here you will find summary of how
scikit-learn
handles multi-class classification for different classifiers.